The Power of Predictive Analytics in Construction
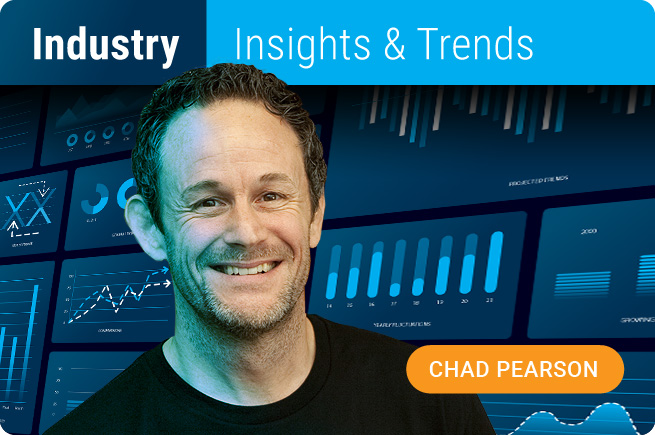
In today’s data-driven world, businesses and organizations are constantly seeking ways to gain insights from the vast amounts of data they collect. Predictive analytics has emerged as a powerful tool to help them make informed decisions, anticipate future trends, and optimize processes. In this blog, we’ll delve into what predictive analytics is, how it works, and its applications in the construction industry.
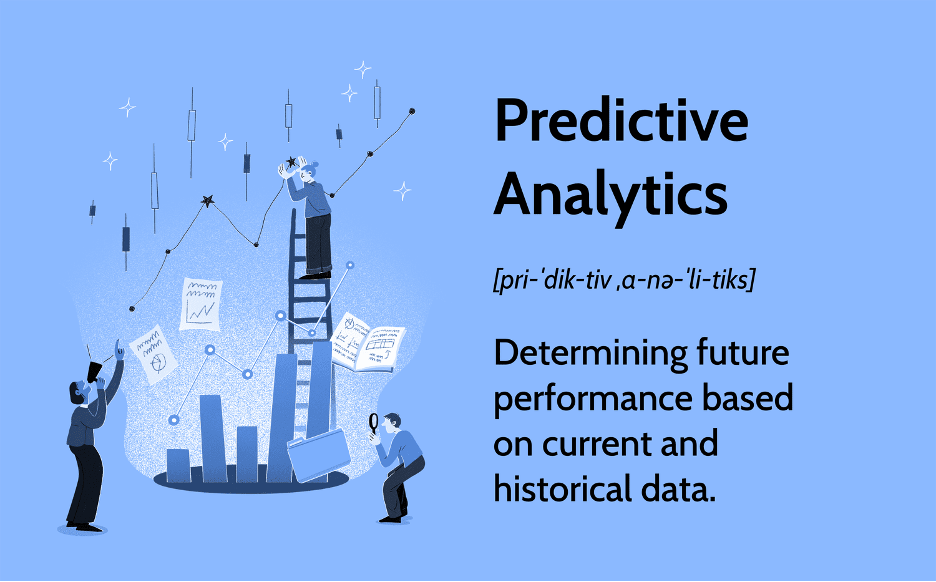
Image source: investopedia.com
What is Predictive Analytics?
Predictive analytics (also known as advanced analytics) is the practice of extracting information from existing data sets to determine patterns and predict future outcomes and trends. It involves using statistical algorithms and machine learning techniques to analyze historical data and make predictions about future events or behaviors.
At its core, predictive analytics seeks to answer the question: “What is likely to happen next?” By leveraging historical data, predictive models can forecast future trends, identify potential risks, and guide decision-making processes.
Imagine you’re a marketing manager trying to forecast customer demand for your product. By analyzing historical sales data, customer demographics, and other relevant factors, predictive analytics can help you predict future sales volumes with a high degree of accuracy. This enables you to make informed decisions about inventory management, marketing strategies, and resource allocation.
How Does Predictive Analytics Work?
Predictive analytics relies on a combination of data, statistical algorithms, and machine learning techniques to generate predictive insights. Here’s a step-by-step overview of how it works:- Data Collection: The process begins with the collection of relevant data from various sources. This data can include historical records, customer demographics, transactional data, social media interactions, sensor data, and more.
- Data Preprocessing: Once the data is collected, it undergoes preprocessing to clean, transform, and prepare it for analysis. This step involves handling missing values, removing outliers, and encoding categorical variables.
- Feature Selection and Engineering: In this step, relevant features (or variables) that are likely to influence the outcome are selected or engineered. Feature selection helps improve the model’s accuracy and efficiency by focusing on the most important variables.
- Model Building: Predictive models are built using various statistical algorithms and machine learning techniques such as linear regression, logistic regression, decision trees, random forests, neural networks, and support vector machines. These models are trained on historical data to learn the underlying patterns and relationships between variables.
- Model Evaluation: Once the model is trained, it is evaluated using validation techniques to assess its performance and accuracy. Common evaluation metrics include accuracy, precision, recall, F1 score, and area under the ROC curve (AUC).
- Deployment and Monitoring: After successful evaluation, the predictive model is deployed into production, where it can generate predictions on new data. It’s essential to monitor the model’s performance over time and retrain it periodically to ensure its effectiveness and relevance.
Types of Predictive Analytics Techniques
Let’s delve deeper into the various predictive analytics techniques.- Regression Analysis: Predicting a continuous outcome variable based on one or more predictor variables. For example, predicting house prices based on factors like location, size, and amenities.
- Classification: Categorizing data into predefined classes or categories. For instance, classifying email messages as spam or non-spam based on their content.
- Time Series Analysis: Analyzing data collected over time to identify patterns and make forecasts. This is commonly used in financial markets to predict stock prices or in meteorology to forecast weather conditions.
- Clustering: Grouping similar data points together based on their characteristics. This can help identify patterns and segment the data for further analysis.
- Neural Networks: A type of machine learning algorithm inspired by the structure and function of the human brain. Neural networks are capable of learning complex patterns from data and making predictions in a wide range of applications.
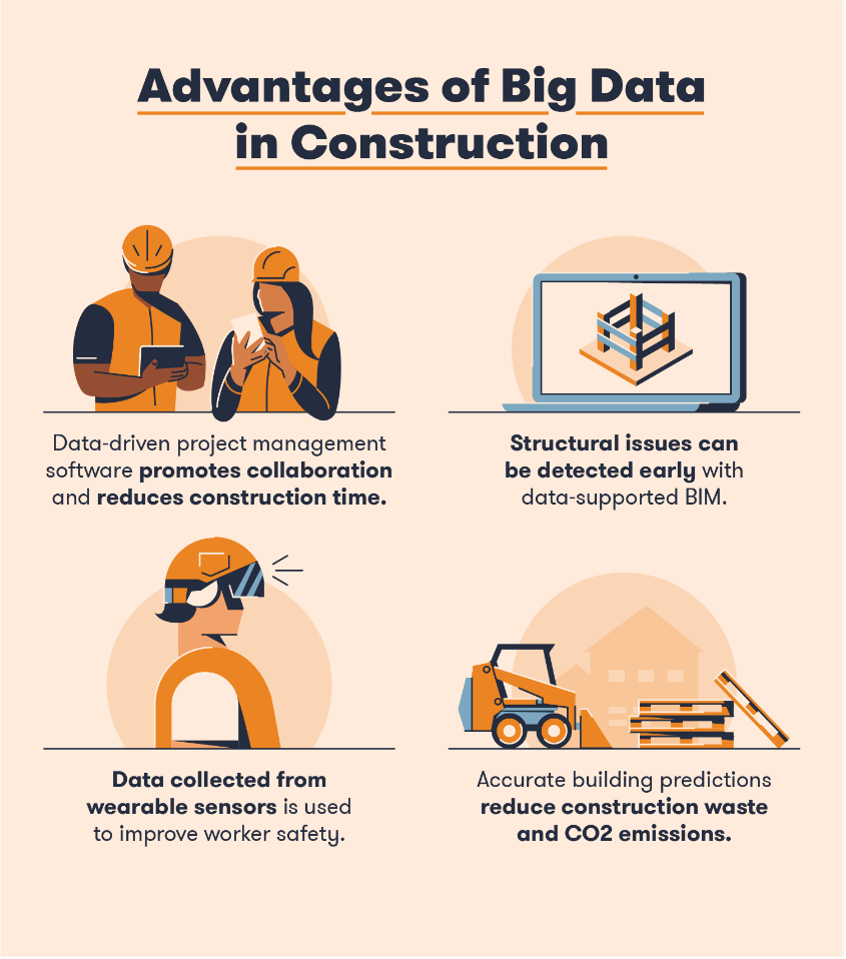
Image source: bigrentz.com
Predictive Analytics in the Construction Industry
Here’s how predictive analytics is making an impact in construction:- Project Planning and Scheduling: Predictive analytics can help construction companies forecast project timelines more accurately by analyzing historical project data, resource availability, weather patterns, and other relevant factors. By identifying potential delays and bottlenecks in advance, project managers can optimize schedules, allocate resources efficiently, and ensure timely project completion.
- Cost Estimation and Budgeting: Enables construction firms to generate more accurate cost estimates and budgets for construction projects. By analyzing historical cost data, material prices, labour costs, and other project parameters, predictive models can help forecast project costs more reliably, reducing the likelihood of budget overruns.
- Risk Management: Helps construction companies identify and mitigate project risks more effectively. By analyzing historical project data and external factors such as market trends, regulatory changes, and geopolitical events, predictive models can assess the likelihood and impact of various risks, allowing project teams to implement proactive risk mitigation strategies.
- Resource Allocation and Optimization: Assists construction firms in optimizing resource allocation, including labour, equipment, and materials. By analyzing project requirements, resource availability, and historical utilization data, predictive models can help identify opportunities for resource optimization, reducing idle time and improving overall productivity.
- Equipment Maintenance and Management: It is used to optimize equipment maintenance schedules and minimize unplanned downtime. By analyzing equipment performance data, usage patterns, and sensor readings, predictive models can forecast equipment failures before they occur, enabling proactive maintenance and reducing costly downtime.
- Quality Control and Defect Detection: Helps construction companies improve quality control processes and detect defects early in the construction process. By analyzing data from sensors, drones, and other monitoring devices, predictive models can identify deviations from expected quality standards and trigger alerts for corrective action, ultimately improving the quality and durability of constructed assets.
- Supply Chain Management: Assists construction firms in optimizing supply chain management processes, including procurement, inventory management, and logistics. By analyzing historical supply chain data, market trends, and supplier performance metrics, predictive models can help forecast demand, optimize inventory levels, and identify opportunities for cost savings and efficiency improvements.
Applications of Predictive Analytics Across Other Industries
Predictive analytics finds applications across a wide range of industries and domains.- Marketing and Sales: Helps businesses identify potential customers, personalize marketing campaigns, forecast sales trends, and optimize pricing strategies.
- Finance and Banking: In the finance sector, it is used for credit scoring, fraud detection, risk management, and stock market forecasting.
- Retail: Retailers leverage predictive analytics to anticipate customer demand, optimize pricing strategies, and minimize inventory costs.
- Healthcare: Enables healthcare providers to anticipate patient outcomes, identify high-risk individuals, optimize treatment plans, and improve patient care.
- Manufacturing: Manufacturers use advanced analytics for predictive maintenance, predicting equipment failures before they occur and optimizing production schedules to minimize downtime.
- Human Resources: HR departments utilize this data for talent acquisition, employee retention, workforce planning, and performance management.
- Insurance: Predictive analytics is employed for risk assessment, underwriting, claims processing, and fraud detection. By analyzing historical data and relevant risk factors, insurers can better predict future losses and set appropriate premiums.
- Sports Analytics: It is increasingly used in sports for player performance analysis, injury prediction, game strategy optimization, and fan engagement.
- Telecommunications: Companies utilize this data for customer churn prediction, network optimization, and capacity planning.
- Logistics: Utilized in transportation and logistics for route optimization, demand forecasting, vehicle maintenance scheduling, and supply chain management.
*Brought to you by Plexxis Software: Offering software solutions for the construction industry that integrates cloud, mobile and on-premise software to improve and enhance team performance.
Share:
New From Plexxis
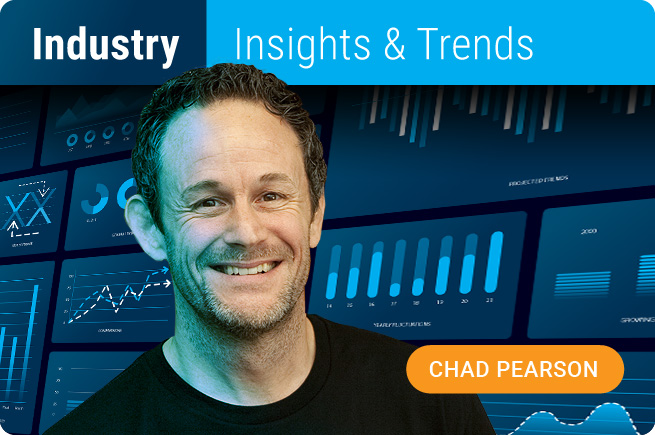
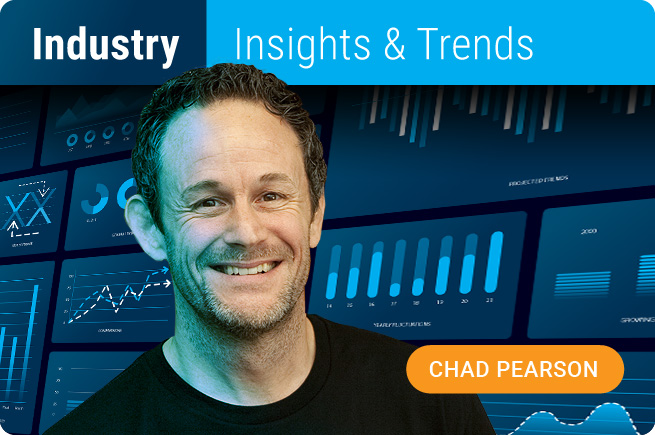
Social Engineering as a Cyberattack Tactic
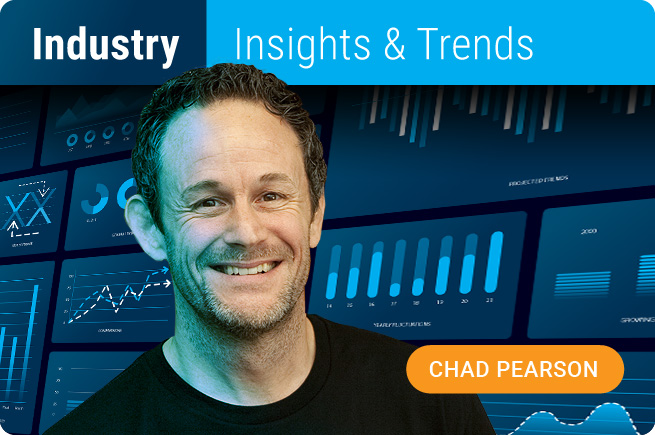
Ransomware Protection: Keep Your Data Safe
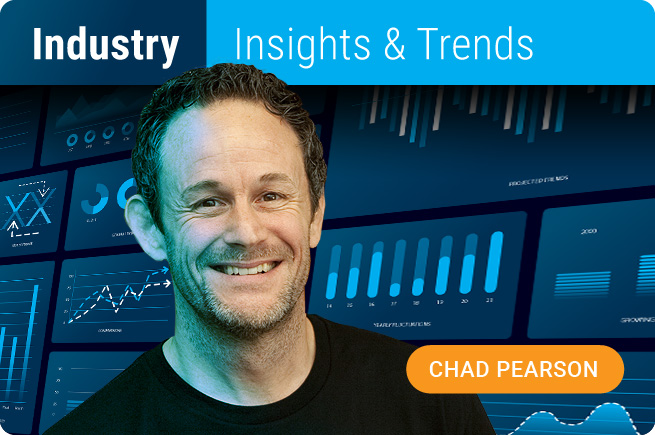